Personal Social Networks and Relapses in Women With Substance Dependence: Application of the Theory of Planned Behavior
Article information
Abstract
Objective
Establishing positive network resources and rebuilding drug free networks are key components of recovery process for women with substance use disorder (SUDs). Theory of planned behavior (TPB) posits that behaviors are determined by behavioral intentions, which are determined by three factors: attitude toward the behavior, perceived behavioral control, and subjective norms. The current study applied TPB to examine whether women’s personal social network characteristics (as subjective norms) were related to intentions towards, and/or a substance use relapse using prospective research design.
Methods
Longitudinal data from 300 American women with SUD who received treatment at three county-funded, women-only intensive treatment programs was utilized for the current study.
Results
Bayesian estimation path analyses indicated that attitude and abstinence self-efficacy at intake were not related to intention at the 6-month follow-up, but abstinence self-efficacy was directly related to substance use relapse by 12 months. Personal social network characteristics, number of treatment related persons in personal social network and sobriety support were significantly associated with intention at 6 months. The interaction between the numbers of treatment related persons and density was found on intention, suggesting that density strengthens the relationship between treatment related persons in network and intention. Intention to use substance was associated with relapse to substance use behavior.
Conclusion
Our findings support that personal social network characteristics can be considered as subjective norms in the TPB model. Personal social network interventions focusing on rebuilding consistent social norms against drug-using behaviors may decrease intention to use substance, which will lead to abstinence.
INTRODUCTION
Women use substances differently than men. Women are more likely to self-medicate with substance to cope with emotional, relational problems [1]. Although women tend to begin using alcohol and drugs at lower levels than men do, their use escalates to addiction more quickly (“telescoping” effect) [2]. In addition, women are more likely to suffer from substance abuse related problems such as unbalanced sex hormones, panic attacks, depression, and overdose, may have more drug cravings, and more likely to relapse after treatment [3]. In spite of multiple resources available in substance use treatment, relapse to substance use is frequent [4]. Relapse is the recurrence of substance use disorder (SUD) symptoms after a period of reduced substance use [4,5]. While relapse can be a normal part of recovery process, for some substance, it can be very dangerous. Relapse rates may vary with its definition, study population, type of substance, and time since treatment. In general, the relapse prevalence rate for SUD is estimated to be between 40% and 60% [6], with European studies indicating between 40% and 75% for heroin [7] and other illicit drugs [8]. These rates are similar to the rates of relapse for other chronic diseases such as hypertension or asthma [6], supporting that SUD should be treated as a chronic illness that distresses the entire body [6].
Identification of factors associated with relapse risk among women with SUD is critical for the development of appropriate treatment programs for those at risk. Despite substantial research on substance use focusing on individuals, clinical and treatment characteristics in SUD treatment settings, characteristics of substance users’ personal social networks (PSNs) have been under-recognized [9]. In particular, little is known about how the composition, structure and support of women’s PSNs may impact substance use relapse. Women are more influenced by the people in their networks during substance use or recovery than men [10], experience more traumatic events that impact their interactions with their networks [11,12]; and ted to have lower social support for recovery [2]. Women are more likely to have a co-occurring mental disorder, and women with co-occurring mental and SUDs may have more difficulty in accessing and using recovery supports [13], increasing the risk of substance use relapse [14].
Theory of planned behavior and relapse of women’s substance use
The theory of planned behavior (TPB) is a widely used social cognitive model of health-related behavior, including substance use [15,16]. The TPB posits that attitudes, subjective norms, and perceived behavioral control predict intentions to engage in a behavior; and intentions and possibly perceived behavior control predict actual behavior [17]. Actual behaviors are predicted by the individual’s attitude toward the behavior (how favorable or unfavorable the behavior is perceived to be) and by their perception of social norms as regards the behavior (perceived expectations of others regarding the behavior) [17]. In addition, perceived behavioral control (also conceptualized as self-efficacy) is considered to affect behavior directly and indirectly through behavioral intention. The TPB has been widely used in explaining substance use behavior [18-21], indicating that more positive attitudes and perceived norms to substance use, and lower efficacy to refuse substance use are related to greater intentions to use drugs, and those intentions in turn predict substance use behavior [22,23].
Although the TPB is a useful model, over the years, the TPB has been criticized for its poor predictive efficacy, in particular to the inclusions of an insufficient number of variables [24], calling for additional variables to increase the model’s ability to explain a behavior beyond the original variables. Especially the subjective norm has been a weak predictor of intentions [25], with multiple studies suggesting that the normative component within the TPB inadequately account for various ways that social influence can be exerted [26]. Typically, for the last decade social norms are formed by observing and modelling the behavior of family members, peers and friends, and including PSN characteristics may reflect more diverse perspective of subjective norms in the TPB model [27]. In fact, PSN characteristics have been shown to account for substance use behaviors [14,28-30]. Given that women are more influenced by the people in their networks during substance use or recovery than men [10], women’s PSNs could play an important role in shaping subjective norms. However, few studies have examined the role of PSN within the TPB model.
The existing research on the TPB of substance use behaviors is limited by use of cross-sectional or retrospective research designs [31,32], compromising its internal validity. In predicting actual substance use behavior based on the TPB, a cross-sectional survey does not allow researchers to examine actual future behavior. In order to examine the relationship of the factors in TPB model in substance use behavioral change processes, this study utilized data from a prospective longitudinal research design.
Aims of this study
The present study examined the application of the TPB to understanding intentions and relapse of substance use over a 12-month period in a sample of women with SUD. Women’s PSN variables were utilized to represent subjective norms. The primary goal of this study is that whether PSN characteristics can be considered as subjective norms in the TPB model. As illustrated in Figure 1, we hypothesized that 1) attitude and abstinence self-efficacy would be directly related to substance use intention; 2) abstinence self-efficacy would be directly and indirectly related to substance use relapse via substance use intention; 3) PSN factors as subjective norm would be directly related to substance use intention; and 4) intention would be related to substance use behavior.
METHODS
Sample and research design
In the present study, the sample was women who received substance abuse treatment from three county-funded, women-only treatment programs in Northern Ohio. Women were eligible to be included in the study if they were 18 years of age or older, had been in treatment for at least one continuous week, and had a diagnosis of substance dependence defined as a Diagnostic and Statistical Manual of Mental Disorders, 4th edition (DSM-IV) within the past 12 months of entry into the study for at least one substance, including alcohol. Participants with a known diagnosis of schizophrenia or who were taking medication prescribed for a major thought disorder were excluded [33]. The study was reviewed and approved by the Case Western Reserve University Internal Review Board (#20090215) for the protection of human subjects.
The study protocol, including signed written and informed consent at each follow up interview, was approved. Participants received a gift card ($35) at each interview, plus reimbursement for travel expenses. A Certificate of Confidentiality was obtained from the Department of Health and Human Services to protect against the release of drug-related information. The data were collected over the course of a year: at one-week post treatment intake, and at 1, 6, and 12 months post-intake between October 2009 and May 2012. A total of 377 women were enrolled in the study, and 372 were eligible for follow up (5 were deceased, withdrew or too ill).
Overall retention was 93% at 1 month and 81% at both 6 and 12 months. The present analysis includes 300 women, 80.6% of those eligible for follow-ups, who provided substance use data at 12 months. Those lost to follow up were more likely to report higher trauma symptoms at intake; no difference was found in race, age, dual disorder status, homelessness, legal involvement, employment, number of substances dependence, and duration of SUD [34].
Complete demographic and clinical characteristics for the sample at intake are presented in Table 1. Study participants (n=300) had an average age of 36.7 years (standard deviation [SD]=10.6, range=18–63). Over 60% of participants were African American, and 44.1% had less than high school education. Two-thirds of the women had never married, and nearly three-fourths qualified as low income, receiving food stamps or/and welfare assistance. The women had 2.8 (SD=2.2) children on average. Over 40% of women were living in shared, double-up or temporary housing, with 43.3% reporting periods of homelessness. Approximately three-fourths were dually diagnosed based on the criteria in the DSM-IV, with over 50% of the women diagnosed with multiple SUDs.
Measures
Since there is no standard scale offered to measure TPB constructs, the TPB measures were created for this study in accordance with the guidelines from Ajzen [18]. Descriptive statistics of these variables are shown in Table 2.
Relapse to substance use behavior (12 month)
Substance use, the outcome behavior variable, was assessed using 12 items inquiring about the participants’ behavior in the past six months. Using wording from the Individual Assessment Profile [35], respondents were asked at the 12 month interview, whether and how often they had used any of 12 substances (e.g., marijuana/hash, cocaine/crack, opiates, heroin, depressant, alcohol) to get high or to feel better since the previous interview. Respondents reported their frequency of substance use in the past 6 months as never (0), 1–3 times per month (1), 1–6 times per week (2), and daily (once or more times a day) (3). The variable was recoded as a dichotomous variable (yes/no) indicating any substance use by 12-month post treatment intake due to skewed distribution. Approximately 30% of the participant were in relapse of substance use at 12 months.
Intentions (6 month)
Since intentions may be altered over time, it is suggested to measure the intention as closely as possible to the behavioral observation to obtain an accurate prediction [18]. In this study, at 6-month post treatment intake, a single question was used to measure intentions for substance use: “How many times in the past 30 days have you attended an AA/NA/CA or any other 12 step meeting?” Respondents reported their intention of substance use retrospectively as never (0), 1–10 times (1), 11–20 times (2), 21–30 times (3), 31–40 times (4), 41–50 times (5). The variable was reverse-recoded, with higher scores indicating increased intentions to use the drugs or alcohol. Since the measure of behavioral intention to use substances is the frequency of attending support group meetings, this behavior is a proxy for behavioral intention.
Attitudes (one week)
The attitude toward substance use was measured by adapting 9-item scale originally proposed by Joe et al. [36]. Items were reconstructed to measure attitude toward recognized problem of substance use (e.g., you will give up your friends and hangouts to solve your substance use problems). Items are rated on a 5-point scale (1=strongly disagree to 5=strongly agree), producing a possible range 9–45. Higher scores indicate greater negative attitudes against substance use behavior. The internal consistency of the attitude measure was 0.81.
Perceived behavioral control (one week)
Abstinence self-efficacy was assessed using the Drug Abstinence Self Efficacy Scale, which was modified after the Alcohol Abstinence Self-Efficacy Scale [37]. An example item is “How confident are you right now that you would not use alcohol or drugs: If you were feeling depressed or lonely.” Each participant rated herself on a 5-point Likert scale of confidence to abstain from alcohol and drugs across 20 different highrisk situations (1=not at all confident to 5=extremely confident; range 20–100), with higher scores indicating greater confidence. The Chronbach’s α for the total score=0.97 for this study.
Subjective norms (6 month)
PSN characteristics, as subjective norms, were collected with computer-assisted interview using Egonet software [38,39] by asking each respondent(alter) 1) 25 alters (e.g., partner/ex-partner or spouse/ex-spouse, family member/relative, my birth child or child I am raising, from work or school, from religious group or organization, professional helper, or peers from treatment program including Alcoholics Anonymous and Narcotics Anonymous) with whom they had had any type of contact in the previous 6 months; 2) information about characteristics of those alters; and 3) how each unique pair of alters was likely to be connected to each other. As changes in network characteristics during and post treatment [34], measurement at six months was considered more appropriate than the one at intake. Three items were designed to measure subjective norms toward substance use: network composition (e.g., relationship with respondents), network sobriety support (e.g., the number of people providing sobriety support), and network structure (e.g., how the network members were arranged around the respondents).
Network composition was assessed by the number of treatment related network persons, including professionals of substance use treatment and peers from treatment and 12-step programs, with higher scores indicating more positive social norms against substance use in her network (range from 0 to 25). Network sobriety support was assessed using the number of network persons providing sobriety support (e.g., “give you support to stay clean”) almost always (range from 0 to 25). The higher scores indicate greater perceived sobriety support to the respondent. Network density assesses the extent of cohesiveness, computed as the ratio of the number of present ties divided by all possible ties among network members. Higher scores indicate more densely connected network among network member (range from 0 to 1). Since the influence of treatment related persons and sobriety support on intention may vary depending on the extent of cohesiveness [40], two interaction terms, density by network composition and density by network sobriety support, were included to explore their relationships with substance use intention.
Data analysis
Preliminary analyses
Bivariate correlations were conducted using SPSS 24 (IBM Co., Armonk, NY, USA) to examine the relationships between all study variables. Multicollinearity was assessed by examining variance inflation factor (VIF); no multicollinearity problems were detected as evidenced by low VIF values (the highest VIF value was 1.25).
Structural equation model
Structural equation model (SEM)-based path analysis was conducted via AMOS 22 [41] to examine substance use behavior specified in Figure 1. Due to the use of a dichotomous variable (substance use coded as 1 and non-use coded as 0), Bayesian SEM estimation employed Makrov Chain Monte Carlo algorithm. This process automatically rescales a binary variable to run a probit model. Bayesian SEM does not provide the familiar chi-square index of model fit nor does it offer modification indices for valuable model refinement. Instead, posterior predictive P (PPP) values [42] are provided to indicate model fit. PPP values and their interpretations differ from Fisher’s [43] traditional p-value and established threshold. Model fit is deemed good the closer the PPP is to 0.5 [42], with values closer to 1 and 0 (0.95 and 0.05 thresholds) indicating poorer fit. Thus, according to Muthén’s [44] recommendation, we assessed models for goodness of fit using PPP values and obtained Bayesian coefficient estimates. Bayesian statistics report a credible interval (CI), instead of confidence interval. A CI is a range within which an unobserved parameter value falls with a particular probability and in the domain of a posterior probability distribution [45]. Missing data were modeled using full-information maximum likelihood, which uses all available information from the observed data. Because the level of missing for most variables was small and/or did not appear to be systematic, the assumption of missing at random was considered plausible [46].
RESULTS
Table 2 presents the correlations for all study variables included in the model. The outcome variable, relapse to substance use behavior, was associated with abstinence self-efficacy (r=-0.173, p<0.01), sobriety support (r=-0.167, p<0.01), and intention to use substance (r=0.179, p<0.01), consistent with hypothesis about the direct effects of the abstinence selfefficacy on the relapse to substance use behavior. Although attitude and treatment related persons were not significantly correlated with the outcome, variables correlated with intention to use substance were correlated with the outcome. Overall, these correlations followed the expected pattern.
Path analysis was performed to test the hypothesized model. As presented in Figure 2, attitude and abstinence self-efficacy at intake and subjective norms at 6-month were specified to predict intention to use substance at 6 months, which in turn was modeled to predict relapse to substance use behavior at 12 months. In keeping with Ajzen’s work [18], abstinence self-efficacy also was modeled to have a direct effect on behavior. Bayesian estimation indicated PPP value 0.36, indicating a good model fit [42]. Only standardized coefficients are reported (Figure 2).
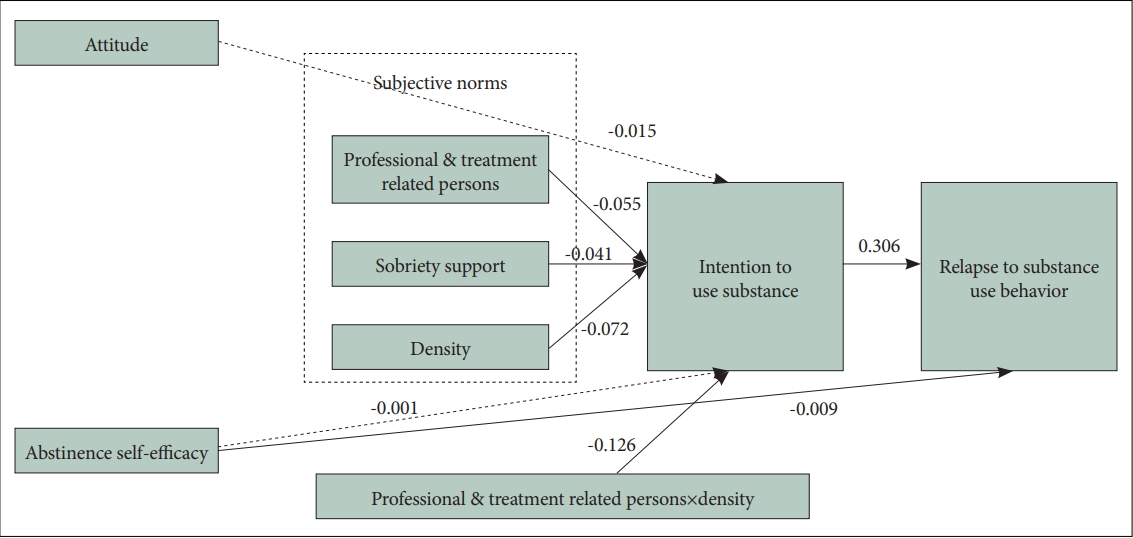
Path diagram for predicting relapse to substance use over the 12-month period. Standardized path (beta) coefficients (regression weights). Path coefficient is significant at the 95 percent credible interval. The unbroken lines represent significant standardized coefficient in the model.
Attitude was not related to intention to use substance (β=-0.015; 95% CI, -0.033, 0.004). Although abstinence self-efficacy was not related to intention to use substance (β=-0.001; 95% CI, -0.006, 0.006), it was related to relapse to substance use behavior (β=-0.009; 95% CI, -0.016, -0.001).
Regarding subjective norm factors, greater number of treatment related persons (β=-0.055; 95% CI, -0.085, -0.024) and greater sobriety support (β=-0.041; 95% CI, -0.070, -0.013) were related to decreased intention to use substance. Although, the density was not significant (β=-0.072; 95% CI, -0.590, 0.448), its interaction term with number of treatment related persons on intention to use substance was significant at the 95% CI of (-0.240, -0.013), with higher density strengthening the relationship between treatment related persons in network and intention. Namely, the more cohesive network with more number of treatment related personale, the less intention to use substance. No other significant interaction (i.e., sobriety support by density) was found. Greater intention to use substance was related to a higher likelihood of relapse to substance use behavior (β=0.306; 95% CI, 0.140, 0.484). The estimated model accounted 11% of the variance in the relapse to substance use behavior.
DISCUSSION
The current study examined the utility of the extended TPB to explain relapse to substance use behaviors over a 12-month period, investigating whether PSN factors as subjective norms affect intentions. Attitude and abstinence self-efficacy at intake were not related to intention to use substance at the 6-month follow-up, but abstinence self-efficacy was directly related to relapse to substance use at the 12-month follow-up. PSN factors, number of treatment related persons and sobriety support available in PSN, were related to intention. Although density was not related to intention, its interaction with the number of treatment related persons explained intention. Namely, density strengthened the negative relationship between the number of treatment related persons in network and intention to use substance. The more densely connected network among network member, the less intention to use substance. Intention to use substance at 6 months was the strongest factor of relapse to substance use behavior at 12 months.
Although the TPB had explained a wide range of health-related behaviors such as drinking, smoking, drug abuse, and aggressive behavior [18,47], the TPB was considerably less predictive of behavior when a longitudinal research design, rather than a cross sectional design, was employed. The present study demonstrated the utility of the TPB in understanding relapse of substance use in the context of longitudinal follow-up, providing additional support for the TPB.
The present study also demonstrates the role of additional normative variables such as the PSN component, structure, and support and the importance of considering interactions among normative variables in the TPB. The subjective norm construct has been generally a weak predictor of intentions [25], calling for a need to expand the normative component [19]. The current study has shown that, consistent with previous findings [40,48,49], having more treatment related persons and more sobriety support in their network are associated with decreased substance use intention. Subjective norms, operationalized as PSN characteristics, may increase the explanation of intention over and above the other TPB variables. Sobriety support and treatment related persons in network were associated with decreased intention for the substance use; and intention was related to less likelihood of substance use. Although density moderated the impact of treatment related persons on intention, it did not moderate the impact of sobriety support on intentions. Higher density supported recovery intention when the network had many helpful persons physically surrounded in the network [9]. Sobriety support from a qualified professional counselor or treatment related persons may provide more concrete and informative support than friends or family members. Given the particularly strong association between PSN variables and intention to use substance, PSN should be a target for prevention and intervention efforts.
Relapse occurred among 30% of the current sample by the 12-month post treatment intake, comparable to that reported in a recent study using a sample of 607 patients with SUD admitted to an inpatient SUD treatment center (relapse rate=37% of the sample by three-month follow-up) [4]. Although direct comparisons of relapse rates between studies are problematic because of the diversity of treatment settings, sample populations, and various follow-up intervals and definitions of relapse, our findings indicate that improving abstinence selfefficacy and PSN, the two main constructs of the TPB, could be promising targets for maintaining abstinence.
There are still a few limitations to the current study. The generalizability of the present study is limited because of the use of a convenience sample of low income, inner-city women served by county service systems. However, given the prevalence of substance use behavior in this population, it is clearly different cultural and ethnic sample in need of further study. Although the current study employed a prospective design, the follow-up period was limited to 12 months; future studies should incorporate a longer follow-up to further examine the utility of TPB in longitudinal design. The current study also relied on the use of a self-report survey, subject to recall bias, and memory distortion. The self-report measure may be responsible for the lower release rate (30%) compared with other studies. Finally, future research should also examine the role of social network services (SNS) and online based cybercommunity as subjective norms.
In conclusion, our findings support that PSN characteristics can be considered as subjective norms in the TPB model. PSN interventions (e.g., continuous professional support and building a cohesive network that consistently supports sobriety) may decrease intention to use substance, which will lead to the reduction of relapse.
Notes
Availability of Data and Material
The data that support the findings of this study are available on request from the corresponding author. The data are not publicly available due to privacy or ethical restrictions.
Conflicts of Interest
The authors have no potential conflicts of interest to disclose.
Author Contributions
Conceptualization: HyunSoo Kim, Meeyoung O. Min. Data curation: HyunSoo Kim, Meeyoung O. Min. Formal analysis: HyunSoo Kim, Meeyoung O. Min. Funding acquisition: HyunSoo Kim, Meeyoung O. Min. Investigation: HyunSoo Kim. Methodology: HyunSoo Kim, Meeyoung O. Min. Project administration: HyunSoo Kim, Doug Hyun Han. Resources: HyunSoo Kim, Doug Hyun Han. Software: HyunSoo Kim. Supervision: Meeyoung O. Min, Doug Hyun Han. Validation: Meeyoung O. Min, Doug Hyun Han. Visualization: HyunSoo Kim. Writing—original draft: HyunSoo Kim. Writing—review & editing: Meeyoung O. Min, Doug Hyun Han.
Funding Statement
This research was supported by National Institute on Drug Abuse R01DA022994 and the Dongguk University Gyeongju Research Fund of 2021.
Acknowledgements
We thank Dr. Elizabeth M. Tracy for the mentorship on this project.