Higher Fat-Related Body Composition Measurement and Lower Resting-State Inter-Network Functional Connectivity of APOE ε4 Carrier in Mild Cognitive Impairment Patients With Aβ Deposition
Article information
Abstract
Objective
We aimed to evaluate the impact of interaction between APOE ε4 carrier status and body composition measurements on intra- and inter-regional functional connectivity (FC) in mild cognitive impairment (MCI) patients with Aβ deposition.
Methods
MCI patients with and without APOE ε4 allele (carrier, n=86; non-carrier, n=95) underwent neuropsychological battery, resting-state functional magnetic resonance imaging scans, positron emission tomography scans with [18F]flutemetamol, and bioelectrical impedance analysis for measuring body composition. We employed a priori defined regions of interest to investigate the intra- and inter-network FC profiles of default mode network (DMN), central executive network (CEN), and salience network.
Results
There was a significant interaction of APOE ε4 carrier status with body fat mass index, visceral fat area, and waist-hip circumference ratio for inter-network FC between DMN and CEN, contributing higher fat-related body composition measurements in the APOE ε4 carrier with lower DMN-CEN FC.
Conclusion
The present results highlight the detrimental effect of APOE ε4 carrier status on the associations between the fat-related body composition measurements and FC in the MCI patients with Aβ accumulation.
INTRODUCTION
Alzheimer’s disease (AD) is the leading neurodegenerative disease, and the Research Framework for AD diagnostic guidelines proposed in 2018 by the National Institute of Aging and Alzheimer’s Association (NIA-AA) emphasizes the identification of an underlying pathology, such as the deposition of amyloid-beta (Aβ) protein, to diagnose AD [1].
In terms of brain function, three resting-state neural network models are known to play a key role in neurocognition: the default mode network (DMN), the central executive network (CEN), and the salience network (SN) [2]. The utility of identifying functional connectivity (FC) within and between resting neural networks has been repeatedly confirmed as a methodology for assessing the impact of AD pathology on the neurodegenerative process [3]. It has been reported that FC within these resting neural networks is significantly reduced in AD patients, and that FC between neural networks is progressively reduced with increasing Clinical Dementia Rating (CDR) scores [4]. A meta-analysis evaluating changes in FC of neural networks in the mild cognitive impairment (MCI) stage, a prodromal stage for AD, also observed abnormal FC changes in the DMN and suggested that it could be used in combination with other biomarkers to predict AD [5].
In recent years, there has been an emphasis on the significance of metabolic diseases, diet, and physical activity intensity as major risk factors for AD [6]. Body composition, which is associated with these factors, has also received increasing attention. It has been reported that higher body mass index (BMI) are linked to cognitive decline in normal cognition [7-9] and that individuals with an obese BMI have an increased risk of developing dementia in the long term [10,11]. Furthermore, there is some evidence indicating that alterations in BMI among older individuals may result in reduced structural connectivity within the brain, as well as diminished FC within restingstate brain networks [12,13]. However, most studies have evaluated at how BMI, which is measured by weight and height alone, is related to neural networks in older adults. Only a few studies have considered multiple measures of obesity as part of body composition. Additionally, no prior research has explored the connection between key body composition measures and neural networks in the course of AD trajectory.
Among the apolipoprotein E (APOE) subtypes involved in lipid transport in vivo, the APOE ε4 genotype is associated with an increased risk of developing sporadic AD dementia [14,15], increased production of Aβ protein [16], and decreased clearance of Aβ protein [17]. Prior research has shown that cognitive decline in individuals with the APOE ε4 allele is linked to reduced connectivity between DMN-CEN neural networks [18]. Previous studies have also found that high BMI in individuals with the APOE ε4 allele is associated with increased [18F] fluorodeoxyglucose uptake in the posterior cingulate cortex among cognitively intact older adults [19]. However, there have been no studies that have investigated differences in the relationship between body composition measurements and FC of the brain’s neuronal networks in those with and without the APOE ε4 allele during the prodromal stages of AD.
Given the results of previous studies, we hypothesized that the the APOE ε4 carrier status would differentially affect the association between body composition and resting-state network FC. As both obesity-related body composition and the APOE ε4 allele contribute to resting-state brain network FC, we expected that in the APOE ε4 carriers of MCI patients, FC of AD-related resting-state neural networks would be more significantly reduced with increasing obesity-related body composition.
Previous studies often lacked information on Aβ deposition or were limited to cognitively normal older adults, and rarely examined the association between body composition measurements other than BMI and resting-state neural network FC. In this study, we performed Aβ ligand-based positron emission tomography (PET) and comprehensive neuropsychological testing to identify patients with prodromal AD, and explored whether the correlations between key body composition measurements and neural network FC differed significantly by the presence of the APOE ε4 allele in patients with amnestic MCI with Aβ deposition. Additionally, considering the findings of previous studies on the association of body composition with cognitive function and Aβ deposition [7-9], and the influence of the APOE ε4 allele on these variables [16,20], we further evaluated the interaction between body composition measurements and the APOE ε4 carrier status for these variables.
METHODS
Participants
All participants were recruited from the outpatient-based Catholic Aging Brain imaging database who visited the Catholic Brain Health Center at Yeouido St. Mary’s Hospital from October 2018 to December 2020, and were aged 60 years or older and 85 years or younger. Cognitive function was assessed using the Korean version of the Consortium to Establish a Registry for Alzheimer’s Disease Assessment Packet (CERAD-K) [21], a standardized clinical neuropsychological test for the evaluation of dementia diagnosis. Among the CERAD-K items, the scores of the Word List Memory, Word List Recall (WLR), and Word List Recognition items were summed to produce a memory-related score, Total Memory (TM). The CERAD-K total score was calculated by summing the scores of the detailed test items except for the Korean version of Mini Mental Status Examination and the constructed behavior test.
The inclusion criteria were as follows 1) complaint of subjective memory decline beyond that expected for age; 2) CERAD-K WLR test score less than or equal to 1.5 standard deviations below the standardized mean score corrected for age, sex, and education years; 3) CDR score of 0.5; 4) no significant impairment in the ability to perform independent activities of daily living; and 5) positive for Aβ deposition: standardized [18F]flutemetamol (FMM) uptake value ratios (SUVRs) in a global cortical area greater than or equal to 0.62 using the pons as a reference region [22].
Exclusion criteria include alcohol use disorder, drug abuse, head trauma, or psychiatric disorder with a history of taking psychotropic medications (cholinesterase inhibitors, antidepressants, benzodiazepines, antipsychotics); multiple uncontrolled cardiovascular risk factors (uncontrolled arterial hypertension, diabetes, hyperlipidemia, cardiovascular disease including coronary artery disease, arrhythmias, etc.); and evidence of subcortical ischemic changes corresponding to a Fazeka’s scale score of 2 or higher [23]. All subjects underwent [18F]FMM PET within 3 months before and after magnetic resonance imaging (MRI). Procedures for APOE genotyping are described in the Supplementary Material (in the online-only Data Supplement). Given the protective effect of the APOE ε2 gene, 24 we excluded subjects with the APOE ε2 allele. Subjects were categorized as APOE ε4 carriers if they carried at least one APOE ε4 allele and as non-carriers if they did not carry an APOE ε4 allele. The study was approved by the Institutional Review Board of The Catholic University of Korea (SC20RISI0198), and documented informed consent was obtained from all study participants.
Functional MRI data processing
Detailed procedures for structural and functional MRI (fMRI) data acquisition are described in the Supplementary Material (in the online-only Data Supplement). We used the Data Processing Assistant for resting-state fMRI (DPARSF; GNU General Public License, Beijing, China) [25], which is based on Statistical Parametric Mapping (SPM 12; http://www.fil.ion.ucl.ac.uk/spm, Wellcome Centre for Human Neuroimaging, London, England), to preprocess the fMRI images. The preprocessing included slice timing, realignment for motion corrections, spatial registration, normalization, and smoothing. This procedure is demonstrated in detail in our previous study [26] and the Supplementary Material (in the online-only Data Supplement).
[18F]FMM was manufactured, and FMM-PET data were collected and analyzed as described previously [22]. MRI for each participant was used to co-register and define the regions of interest (ROIs) and correct partial volume effects arising from the expansion of cerebrospinal spaces accompanying cerebral atrophy. Static PET scans were acquired from 90 to 110 min after 185 MBq of FMM injection. The semi-quantification of FMM uptake on PET/CT scan was performed by obtaining the SUVRs. The volumes of interest (VOIs) were restricted to gray matter, covering the frontal, superior parietal, lateral temporal, anterior, and posterior cingulate cortex/precuneus regions. These VOIs were also considered in a previous study. The reference region for SUVR calculations was pons. The mean uptake counts of each VOIs and reference region were measured on the preprocessed image. A regional SUVR was calculated as the ratio of each cortical regional mean count to the pons mean count (SUVRPONS). The global cortical average (composite SUVR) was calculated by averaging regional cortical SUVRs weighted for size. We used a cutoff for positive neocortical SUVR of 0.62, consistent with cutoff values used in a previous FMM PET study [22]. PET scans classified as positive Aβ accumulation also exhibited normal visual reading. Detailed information on the PET scan and SUVR calculation is provided in the Supplementary Material (in the online-only Data Supplement).
Remote FC analysis: intra- and inter-network connectivity
We used the DMN [27,28], CEN [29], and SN [30], which have been shown to be selectively impaired in the course of AD. The 21 ROIs for DMN, CEN, and SN were defined as spheres of 6 mm radius around the center coordinate. The location-based coordinates of the ROIs have been described in previous studies [4]; Montreal Neurological Institute coordinates for the 21 ROIs are given in Supplementary Table 1 (in the online-only Data Supplement). For each of the three types of resting-state neural networks, intra-network FC strength was defined as the average FC strength of the ROIs within the same neural network, and inter-network FC strength was defined as the average strength of all possible connections between the ROIs comprising each neural network. Further details are provided in the Supplementary Material (in the online-only Data Supplement).
Measuring body composition with bioelectrical impedance analysis
An Inbody770 (Inbody Co., Seoul, Korea) device was used to measure body composition. Body composition analysis by bioelectrical impedance analysis has been proven to be accurate in several studies [31,32]. The analysis works by passing a very small current through the body and measuring the impedance, which is caused by differences in electrical conductivity due to the biological characteristics of the tissue. The measured impedance yields body water, body fat, and muscle mass, which can be used to calculate BMI, body fat mass index (BFMI), and skeletal muscle mass index (SMI), expressed as a ratio to height. In addition, visceral fat area (VFA) and waist-hip circumference ratio (WHR) were measured using ground truth and regression equations according to the manufacturer’s algorithm.
Statistical analysis
All statistical analyses were performed using R software (version 4.0.5; R Foundation for Statistical Computing, Vienna, Austria), jamovi (version 1.6.23; GNU General Public License; https://www.jamovi.org/), and SPM 12. The Kolmogorovsmirnov test in R software was used to assume normality for continuous variables; all data were normally distributed. Differences between APOE ε4 carriers and non-carriers in demographic variables, clinical data, global Aβ deposition, cognitive function, and values of body composition measurements in patients with amnestic MCI were tested using two sample t-tests and chi-squared (χ2) tests. For all statistical analyses, p<0.05 was used to define statistical significance.
In addition, multiple regression analysis was applied to evaluate the influence of the APOE ε4 carrier status on the association between FC and values of body composition measurements in patients with amnestic MCI. The z-transformed correlation values within and between the DEN, CEN, and SN neural networks were used as dependent variables and APOE ε4 carrier status and body composition measurements (BMI, SMI, BFMI, VFA, and WHR) as independent variables, with age, sex, and years of education as covariates. Global FMM SUVRPONS was not included as a covariate given that it did not show a significant correlation with the independent and dependent variables (Supplementary Table 2 in the online-only Data Supplement). Furthermore, we explored the association between FC and body composition measurements in each subgroup stratified by APOE ε4 carrier status, adjusting for age, sex, and education years. Multiple regression analyses were also performed to assess the interaction between body composition measurements and APOE ε4 carrier status on global FMM SUVRpons and neuropsychological test battery scores. Global FMM SUVRpons and TM scores, subdomain scores, and CERAD-K total scores were the dependent variables, and APOE ε4 carrier status and body composition measurements were the independent variables, with age, sex, and years of education as covariates. A p<0.05 was used for statistical significance.
RESULTS
Baseline demographic and clinical data
Table 1 presents the demographic and clinical characteristics of the APOE ε4 carriers and non-carriers. A total of 181 patients were included in the analysis, 86 APOE ε4 carriers and 95 APOE ε4 non-carriers. There were no significant differences in age and sex between the two groups, but the APOE ε4 carriers had approximately 2 years more years of education (p=0.021). In addition, the APOE ε4 carriers had significantly higher global FMM SUVR PONS values compared to the non-carriers (p=0.026). In terms of clinical neuropsychological test battery scores, the APOE ε4 carriers had significantly higher scores on the Korean Verbal Fluency Test (p=0.016) and Boston Naming Test (p=0.010) subscales. No significant differences were observed between APOE ε4 carriers and non-carriers with respect to the following body composition measurements: BMI, SMI, BFMI, VFA, and WHR, and no significant differences were observed between APOE ε4 carriers and non-carriers with respect to the proportion of categories classified as obese based on BMI.
Relationship between body composition measurements and neural network FC by APOE ε4 carrier status
We discovered that the association between FC in the DMN-CEN and BFMI, VFA, and WHR differed significantly based on APOE ε4 carrier status (p=0.047; p=0.032; p=0.038) (Figure 1). APOE ε4 carriers with higher BFMI, VFA, and WHR exhibited lower FC between DMN-CEN (Supplementary Table 3 in the online-only Data Supplement). Furthermore, we identified a significant association between fat-related body composition (BFMI, VFA, and WHR) and internetwork FC in the DMN-CEN in APOE ε4 carriers, but not in APOE ε4 non-carriers (Supplementary Table 4 in the online-only Data Supplement). However, there was no significant interaction with APOE ε4 carrier status for the FC between and within neural networks, except for DMN-CEN. Furthermore, the correlation between body composition measurements and global FMM SUVRpons by APOE ε4 carrier status did not show significant differences. Finally, there were no significant differences in the association between body composition measurements and cognitive function test scores (subdomain scores, TM scores, and total score) according to APOE ε4 carrier status.
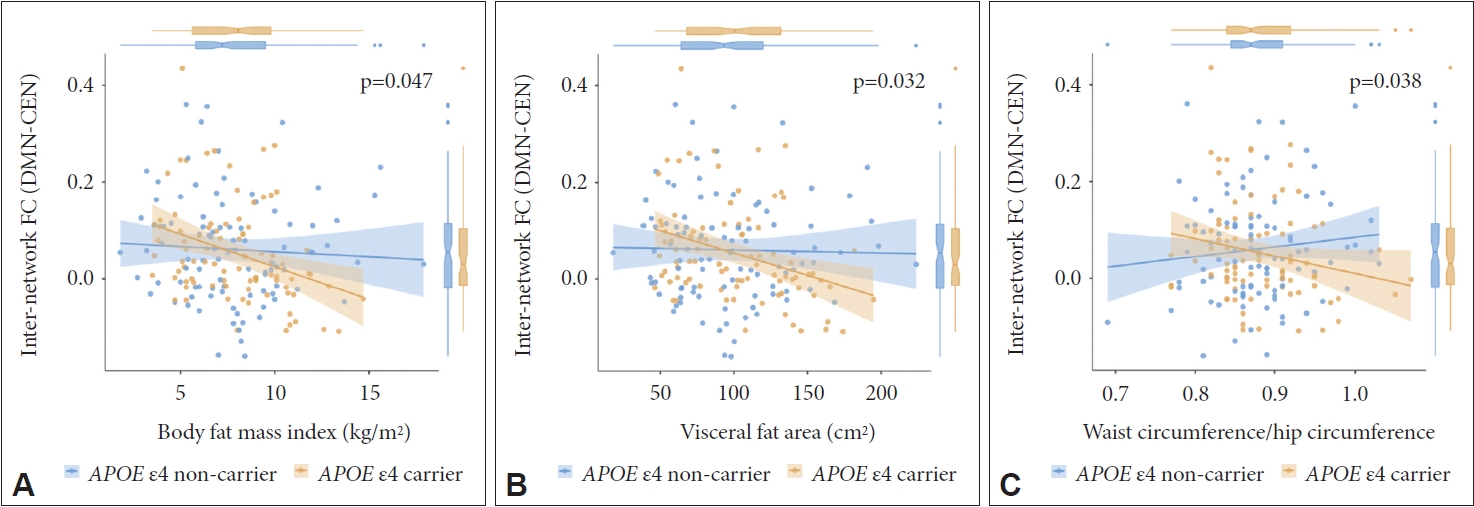
A significant interaction between body composition measurement and APOE ε4 carrier status for inter-network functional connectivity between DMN and CEN in mild cognitive impairment patients with positive Aβ retention. A: Body fat mass index. B: Visceral fat area. C: Ratio of waist circumference to hip circumference. FC, functional connectivity; DMN, default mode network; CEN, central executive network; APOE, apolipoprotein E; Aβ, amyloid-beta.
DISCUSSION
The objective of this study is to evaluate whether the correlation between major body composition measurements and FC within and between AD-related resting-state neural networks varies significantly according to APOE ε4 carrier status in amnestic MCI patients. In order to determine the presence of Aβ deposition, a core pathology of AD, and the level of cognitive function consistent with amnestic MCI, all subjects underwent FMM-PET and a comprehensive neuropsychological test battery.
In the present study, although the BMI, SMI, BFMI, VFA, and WHR did not differ significantly by the APOE ε4 carrier status, the specific body composition subcomponents (BFMI, VFA, and WHR) displayed a significant interaction with the APOE ε4 carrier status for the FC between DMN-CEN in the amnestic MCI patients with Aβ accumulation.
A previous study in cognitively normal older adults has reported that higher BMI was associated with lower FC within the DMN, but that study did not assess FC between neural networks [13]. In addition, prior research including cognitively preserved older adults and MCI patients has reported that the rate of glucose uptake in the posterior cingulate cortex, a key vulnerable region in AD, was significantly higher in obese individuals with APOE ε4 allele, and interpreted the higher posterior cingulate cortex glucose uptake as increased compensatory behavior [19]. The current study evaluated brain function by measuring FC within and between resting-state neural networks and found that higher fat-related body composition in APOE ε4 carriers was associated with lower FC between DMN-CEN neural networks. The APOE ε4 allele is associated with higher cholesterol levels, increased insulin resistance, and an increased risk of type 2 diabetes mellitus, metabolic disease, and coronary artery disease [33-35], all of which can be associated with changes in fat-related body composition, particularly increases in BFMI, VFA, and WHR. In addition, hyperlipidemia, obesity, and the APOE ε4 allele, which are associated with fat-related body composition, have been reported to promote neuroinflammatory processes [36,37], which is one of the major pathological mechanisms of AD [38]. In addition, the neuroinflammatory process has also been shown to impair the FC of resting-state neural networks during the progression of AD [39]. In this regard, the presence of the APOE ε4 allele in individuals at high risk for AD with higher fat-related body composition measurements may have a synergistic effect on brain functional deterioration, facilitating a neuroinflammatory process of AD [40]. Among the neural networks utilized in this study as brain function indicators of AD progression, the CEN requires support from the DMN to maintain cognitive function within the normal range [41], and it has been reported that cognitive decline in APOE ε4 carriers is associated with decreased FC between the DMN-CEN [18]. To gain a deeper understanding of these mechanisms, further investigation will necessitate the utilization of additional brain imaging and blood-based biomarkers in order to evaluate neuroinflammatory factors.
In the current study, the association between body composition measurements and cognitive function did not depend on the presence of the APOE ε4 allele. While most previous studies have found significant results for BMI, this study did not find statistical significance for BMI. The present study demonstrated significant results for BFMI, VFA, and WHR, which are more closely associated with fat than BMI, as BMI does not distinguish between muscle and fat mass. Additionally, while previous studies have focused on individuals with normal cognition, this study focused on patients in the prodromal stages of AD. Finally, the fact that the Korean Society of Obesity uses a BMI for obesity [42] that is 5 kg/m2 lower than the obesity criteria of the World Health Organization [43] may also have affected the discrepancies of the results. In terms of actual BMI criteria, there is not a complete consensus on obesity-related criteria, with Japan proposing 27 kg/m2 as a more appropriate standard [44].
In this study, the association between Aβ deposition and body composition measurements did not differ significantly by APOE ε4 carrier status. Xu et al. [45] have reported a negative correlation between Aβ accumulation and BMI, and previous studies have shown that in APOE ε4 carriers, global Aβ deposition was reduced in those with BMI-based categories of obese compared to overweight and normal individuals [19]. Animal studies [46] have suggested that Aβ deposit stimulates the release of anorexigenic hormones that act on the appetite centers of the hypothalamus, such as leptin, which is associated with a reduction in BMI and weight, but the mechanisms need to be further elucidated. It is possible that no significant interaction was identified in this study because it only included a positive Aβ deposition group to clarify the pathology of AD. However, previous studies included both negative and positive groups of Aβ deposition, allowing for a wider range of Aβ accumulation.
In addition, as this study is a cross-sectional study, by examining the longitudinal effects of changes in body composition on brain function, cognitive function, and Aβ deposition, it will be possible to clarify the role of body composition as an indicator of the intensity of metabolic risk factors for AD. Additionally, evaluating hormonal levels such as leptin and insulin, which function as intermediate factors affecting the pathological process of AD, as well as indicators reflecting the inflammatory process, will help to better understand the role of body composition. In addition, it should be noted that this study solely focused on a specific group of MCI patients with Aβ deposition. However, the effect of AD progression and Aβ accumulation on the research results could not be examined. Therefore, to more accurately evaluate the connection between changes in fat-related body composition and those in brain function modulated by the APOE ε4 allele, it is necessary to conduct further studies that include both control groups and dementia groups caused by AD.
This study investigated whether there was a significant difference in the association between body composition and FC of AD-vulnerable resting-state neural networks, Aβ deposition, and cognitive function in patients with amnestic MCI due to AD based on their APOE ε4 carrier status, which is a representative sporadic AD high-risk gene. By constructing a longitudinal study model and conducting additional studies that encompass a wider range of disease stages, we can achieve a more comprehensive understanding of how metabolic risk factors, which can be easily assessed in daily life, can reflect the neurodegenerative process of AD. Moreover, we would better characterize the role of the APOE ε4 allele, a typical high-risk gene for sporadic AD, in this process.
Supplementary Materials
The online-only Data Supplement is available with this article at https://doi.org/10.30773/pi.2023.0138.
Location of brain regions in resting-state brain networks
Correlation analysis between global FMM SUVRPONS and other variables
Interaction of APOE ε4 carrier status with body composition measurements for inter-network FC between DMN and CEN
Association between body composition measurement and inter-network functional connectivity between DMN and CEN in subgroups stratified by APOE ε4 carrier status
Notes
Availability of Data and Material
The datasets generated or analyzed during the current study are not publicly available due to the Patient Data Management Protocol of Yeouido St. Mary’s Hospital but are available from the corresponding author on reasonable request.
Conflicts of Interest
Yoo Hyun Um and Hyun Kook Lim, a contributing editor of the Psychiatry Investigation, were not involved in the editorial evaluation or decision to publish this article. All remaining authors have declared no conflicts of interest.
Author Contributions
Conceptualization: In Hyeok Choi, Chang Uk Lee, Hyun Kook Lim, Dong Woo Kang. Data curation: In Hyeok Choi, Sheng-Min Wang. Formal analysis: In Hyeok Choi. Funding acquisition: Dong Woo Kang. Investigation: Yoo Hyun Um. Methodology: In Hyeok Choi, Sheng-Min Wang, Dong Woo Kang. Project administration: Dong Woo Kang. Software: Yoo Hyun Um. Supervision: Chang Uk Lee, Hyun Kook Lim, Dong Woo Kang. Visualization: In Hyeok Choi. Writing—original draft: In Hyeok Choi. Writing—review & editing: Sheng-Min Wang, Dong Woo Kang.
Funding Statement
This research was supported by Basic Science Research Program through the National Research Foundation of Korea (NRF) funded by the Ministry of Education (2022R1I1A1A01053710).