Heart Rate Variability Analysis: How Much Artifact Can We Remove?
Article information
Abstract
Objective
Heart rate variability (HRV) evaluates small beat-to-beat time interval (BBI) differences produced by the heart and suggested as a marker of the autonomic nervous system. Artifact produced by movement with wrist worn devices can significantly impact the validity of HRV analysis. The objective of this study was to determine the impact of small errors in BBI selection on HRV analysis and produce a foundation for future research in mental health wearable technology.
Methods
This was a sub-analysis from a prospective observational clinical trial registered with clinicaltrials.gov (NCT03030924). A cohort of 10 subject’s HRV tracings from a wearable wrist monitor without any artifact were manipulated by the study team to represent the most common forms of artifact encountered.
Results
Root mean square of successive differences stayed below a clinically significant change when up to 5 beats were selected at the wrong time interval and up to 36% of BBIs was removed. Standard deviation of next normal intervals stayed below a clinically significant change when up to 3 beats were selected at the wrong time interval and up to 36% of BBIs were removed. High frequency HRV shows significant changes when more than 2 beats were selected at the wrong time interval and any BBIs were removed.
Conclusion
Time domain HRV metrics appear to be more robust to artifact compared to frequency domains. Investigators examining wearable technology for mental health should be aware of these values for future analysis of HRV studies to improve data quality.
INTRODUCTION
Heart rate variability (HRV) is a measurement that evaluates the very small beat-to-beat time interval (BBI) difference produced by the heart. This is different than heart rate, which is the number of beats over a one minute time period. HRV has been used for years as a marker of multiple conditions ranging from cardiac disease to mental health [1-4]. It has gained popularity as prior science has shown HRV to be a marker of the autonomic nervous system [5]. This has opened up the realm of possibilities of this measure to give new insights into areas of medicine that were once thought difficult to examine; especially the case for mental health [1].
HRV has traditionally been measured by chest wall electrocardiography (ECG) [6]. ECG produces the clearest signal with less motion artifact than measurements made on an extremity. Many smartwatches incorporate photoplethysmography (PPG) technology. PPG is an optically obtained plethysmogram that can be used to detect blood volume changes that occur during systole and diastole. PPG comprises a light source and photodetector placed against the skin to measure microcirculatory changes in blood volume that allow for beat to beat detection [7,8]. Smartwatches are a $5 billion industry and estimates show that 1 in 6 adults in the United States owns a smartwatch [9]. The widespread use and incorporation of PPG therefore makes this a tantalizing technology to leverage for medical use. However with the expansion of smart watches and other devices to collect HRV data, motion artifact becomes an issues; especially when worn on the wrist, as motion artifact can lead to errors in picking the heart beat at a consistent point in the cardiac cycle and/or an inability to detect some heart beats. Therefore the reliability of smartwatches to accurately measure pulses for HRV analysis hinges on the ability to account for artifact. Algorithms exist that use motion detection to allow pauses in HRV calculation in real-time, but are not perfect and introduce pauses to the data [10]. HRV relies on very small time changes to calculate the specific time and frequency indices over a longer recording time of minutes, hours and sometimes even days. The questions arise of how many beat to beat intervals one can throw out from a time recording and how much inconsistency in picking the beat at a constant point in the cardiac cycle before the HRV indices are unreliable. The objective of this study was to determine what threshold of removal and what threshold of beatpicking error results in significant HRV differences. This produces a foundation for future analysis to guide the maximum amount of artifact that can be removed from an ECG tracing without altering your overall HRV calculations.
METHODS
This was a sub-analysis from a prospective observational clinical trial registered with clinicaltrials.gov (NCT03030924). This study had Institutional Review Board approval (OHSU 16864). In this trial, adolescents were enrolled for acute suicidality and they wore study wrist devices that utilized PPG to calculate HRV metrics. Subjects wore the study devices for 7 days. Because of difficulty obtaining long-duration sets of clean data, the data were separated into 1 minute sections of good PPG waveform tracings in order to calculate the HRV time and frequency metrics. The most common scenarios encountered due to artifact with PPG tracings are that the peak of a heartbeat is not selected at a consistent point in the cardiac cycle or motion affects multiple beats to the point a beat can’t be reliably detected and a section of data needs to be removed. A cohort of 10 random subject one-minute sections was included in this study. For each one-minute section, the data were artificially manipulated to simulate the two most common non-physiological artifact scenarios that HRV systems generate, as mentioned above. The first set of tests simulated scenarios where the BBI is incorrect because beat picking occurs at an inconsistent point in the cardiac cycle. This might be due to imperfect beat picking algorithms, noise or interpolation. If a given beat is picked late, this has the effect of increasing the selected BBI while decreasing the next BBI. In this set, two variations were applied to all data. First, the sample on which the beat was picked was randomly shifted by a designated number of samples between 0 and 24, either forward or backward in time. In the second variation, the sample on which the beat was picked was delayed for every-other sample between 0–24. This represents a scenario where beat picking might have a time-bias for picking some beats. HRV metrics were calculated with no shifted beats and then when every-other beat detection was delayed between 0–24 samples.
The second set of tests simulated sections of data where one or more beats need to be entirely removed. When a single detected heart beat is missed, this has the effect of either removing the two BBIs that are derived from that heart beat or creating error in the time at which the beat is picked, for example when linear interpolation is used to select a time for the missing beat. HRV metrics were calculated without any removed BBIs and then when between 2–36% (1 to 24 beats) of BBIs were removed. In the first manipulation, successive BBIs were sequentially removed and in the second variation, BBIs were randomly removed up to the maximum described above.
HRV metrics were calculated for each study manipulation. Time domain metrics include Root Mean Square of Successive Differences (RMSSD); Standard Deviation of NN intervals (SDNN) where NN refers to “next normal” beat intervals; mean number of times in which the difference in NN intervals is greater than 50 milliseconds (pNN50) and frequency domain metrics include the power in the high frequency (HF) and low frequency (LF) components. We set the threshold for what was considered a clinically significant change in HRV metrics as a 5% change in mean absolute percent difference.
Instrumentation
The optical signal was created and detected by an OSRAM SFH 7070. This combination optical source and detector includes two green (635 nm) photodiodes which flank the photodetector. The current through the optical source is controlled by a Texas Instruments AFE 4,044, which also detects the output of the photodector at 300 Hz using a 23-bit sigma delta converter with ambient light cancellation. After detection, the data were filtered to remove baseline wander, such as that which occurs due to respiration, and the beats were detected using the Automatic Multiscale Peak Detection (AMPD) algorithm [11]. All waveforms and beat selections were manually reviewed.
RESULTS
10 random subject waveforms were included in the study, each with a 1 minute tracing. Specific age and gender was not possible from the de-identified data. The PPG curves were reviewed and selected as they were found to be free of artifact.
RMSSD
Mean absolute percent difference stays below 5% when beats were randomly shifted by 5 samples and when every other beat was shifted to the right up to 5 samples (Figure 1). Increasing every-other-other BBI (decreasing the interleaved BBIs) has more effect than random changes in the BBI as detailed in Figure 1. Mean absolute percent difference was below 5% when a percentage of beats are removed up to 36% of beats. This was true for both random removal and consecutive removal.
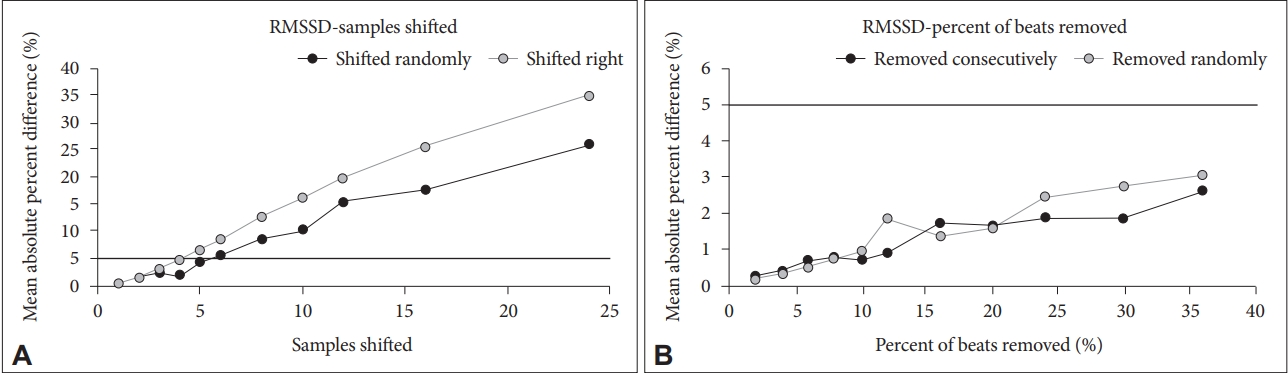
Mean Square of Successive Differences (RMSSD). A: For shift, mean absolute percent difference stays below 5% until about 5 samples for a random shift and about 5 samples for a shift to the right. B: For percent of beats removed, mean absolute percent difference is always below 5% (up to 36 % of beats removed). It's about the same for removal randomly versus consecutively.
SDNN
Mean absolute percent difference stays below 5% when shifted until 3 samples have been altered (Figure 2). This was true for both a random shift versus a shift only to the right. Mean absolute percent difference is always below 5% when beats removed up to 36% of beats. This was true for random beat removal and consecutive removal.
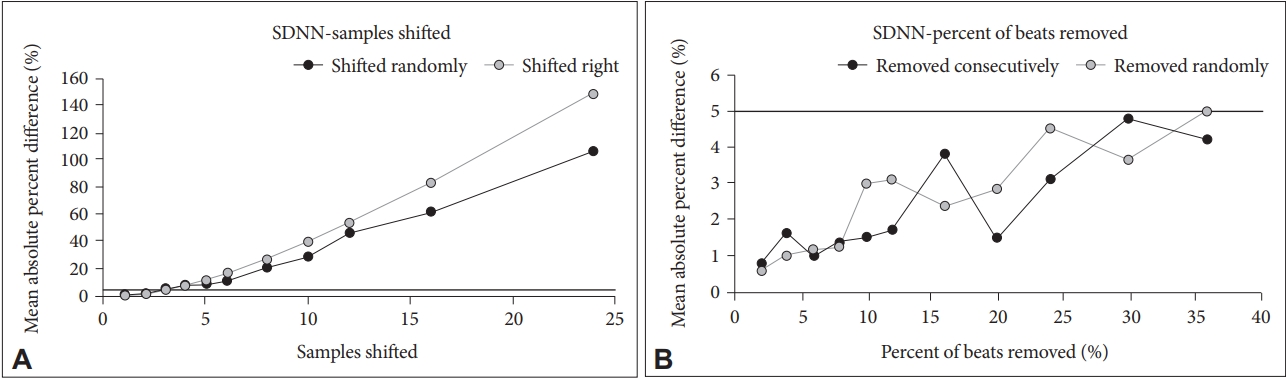
Standard Deviation of NN intervals (SDNN). A: For shift, mean absolute percent difference stays below 5% until about 3 samples and then dramatically increases. It's about the same for random shift versus right shift. B: For percent of beats removed, mean absolute percent difference is always below 5% (up to 36% of beats removed). It's about the same for removal randomly versus consecutively.
pNN50
pNN50 is very sensitive to beat shifting. Any amount of shifting, whether random or right only, pushes average absolute percent difference to more than 5% (Figure 3). Mean absolute percent difference is below 5% until 4% of BBI were removed. This is true for both random and consecutive beat removal.

Mean number of times in which the difference in NN intervals is greater than 50 milliseconds (pNN50). A: PNN50 is very sensitive to shifting. No amount of shifting keeps the average absolute percent difference less than 5%. B: For beats removed, mean absolute percent difference is below 5% until about 4% of beats for randomly or consecutively removed beats.
LF
LF if very robust to shifting BBI right. Mean absolute percent difference stays below 5% until 6 random beats were shifted and then increased (Figure 4). There was no amount of shift to the right that makes the mean absolute percent difference rise above 5%. LF is very sensitive to beat removal with the mean absolute percent difference only staying below 5% when 2% of beats are removed. This is true for both random and consecutive beat removal.
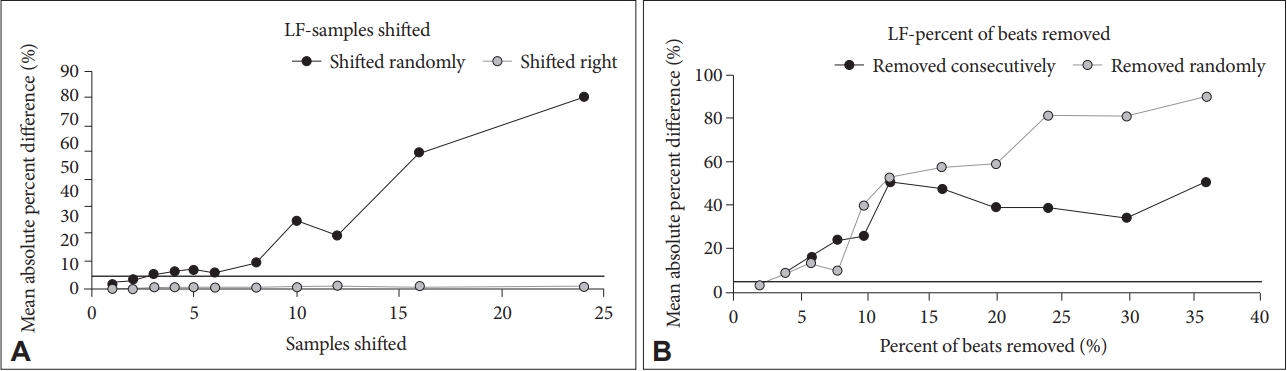
Low Frequency (LF). A: For shift, mean absolute percent difference stays below 5% until about 2 samples, hovers around 5% until about 6 beats, and then dramatically increases. For a right shift, no amount of shift makes the mean absolute percent difference rise above 5%. LF if very robust to shifting samples right. B: For beats removed, LF is very sensitive with the mean absolute percent difference staying below 5% for only 2% of beats removed. It’s the same regardless of random versus consecutive removal.
HF
Mean absolute percent difference stays below 5% for beat shifting until 2 samples of random shift (Figure 5). For a right shift, the mean absolute percent difference remains below 5% until 8 samples shifted. HF is very sensitive to random beat removal with the mean absolute percent difference always being greater than 5% with any amount of beats removed. However, for beats removed consecutively the mean absolute percent difference stays at or below 5% until 8% of beats removed.
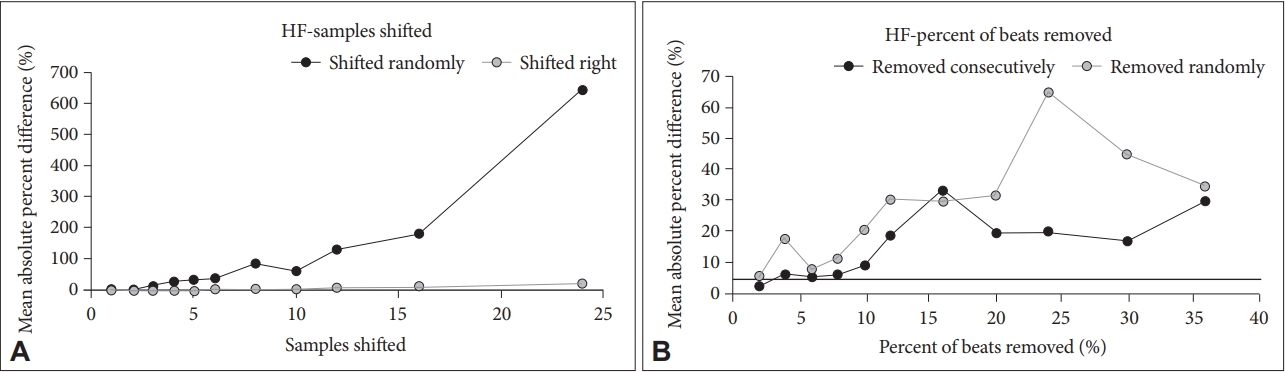
High Frequency (HF). A: For shift, mean absolute percent difference stays below 5% until about 2 samples for a random shift. For a right shift, it mean absolute percent difference remains below 5% until about 8 samples shifted. B: For beats removed randomly, HF is very sensitive with the mean absolute percent difference always being greater than 5% with any amount of beats removed. For beats removed consecutively, that hovers around 5% mean absolute difference until 8% of beats removed.
DISCUSSION
HRV is a measure that has been around for decades and has promise in multiple medical conditions. Recent advances in wearable technology have expanded the application of these measures to improve recognition of chronic disease. However, the accuracy of measures has been an issue with wearable technology, particularly on physically active individuals. The artifact that can be introduced due to movement of wrist-worn devices in particular can create difficulty selecting a consistent point in the PPG waveform that is critical to calculate HRV metrics. This study provides a foundation for threshold values one may consider when reviewing PPG data that has small amounts of artifact.
One interesting finding from this study was the effect shifting the time at which a beat was picked has on HRV analysis compared to removing entire beats. Both of these manipulations were performed to evaluate whether it is better to delete beats or to include a beat that has an error in the time it was picked as a compensation method for artifact. Many times, a small amount of artifact or imperfect beat picking algorithms may cause uncertainty in selecting a consistent point in the waveform resulting in detection shifted by a small number of samples. This data suggests that shifting beats may have more effect on the HRV metrics than removing beats. For RMSSD and SDNN, one could remove up to one-third of the beats in the data without changing the overall HRV by 5% or more. However if beats were shifted by 3–5 samples it quickly altered the HRV metrics to a significant degree. Assuming the BBIs comprise a normal distribution and knowing that RMSSD and SDNN include averaging, (both divide by the number of samples) it is not surprising that removing beats has little effect on these metrics.
One recent study evaluated the effect of missing beat intervals on HRV metrics [12]. This study used ECG recordings over 5 minutes and then wrist-worn PPG over a 24 hour period. Their findings were similar to this study, showing the RMSSD and SDNN were the more robust metrics to removing beats from analysis. One interesting finding their study had was the inability to calculate many HRV metrics from the wrist worn PPG data due to artifact. Our study utilizes multiple 1-minute segments rather than a continuous 24 hours. Depending on the underlying medical condition or state one is hoping to monitor, multiple one-minute segments over a 24 hour period may be suitable for analysis rather than trying to obtain a clean 24 hours of continuous waveforms. Prior review papers have suggested the ability to calculate HRV metrics with data in this shorter duration window with time domains more likely than frequency domain metrics to be accurate in shorter periods of waveform data [6]. This data suggests that it may be better for HRV analysis of RMSSD and SDNN to remove beats with difficult-to-identify peaks rather than interpolating or selecting a beat time that is incorrectly shifted by more than 5 samples (16 ms).
Wrist-worn PPG introduces a novel method for detection of pulses and BBIs with continuous monitoring. Prior studies have attempted to compare PPG to ECG. One study showed that artifact from wrist-worn PPG compared to ECG can significantly affect specific parameters [13]. This study found that the pNN50 measurement was approximately 10 times more affected than SDNN. Our study had similar findings of SDNN being a less affected measure with beat removal and shifting. PPG has been shown in multiple studies to have accuracy comparable to ECG when patients are less active and range from wrist devices to ear lobe technology [14,15]. This expands their utility, but motion artifact is an issue. Algorithms have been developed to account for motion artifact with PPG acquisition that show promise in HRV analysis [16]. A recent systematic review included 18 studies of wearable technology for HRV measurement [17]. This review found that in stationary situations, the agreement between ECG and wrist worn technology is good to excellent. However as non-stationary conditions increase, HRV accuracy significantly decreases. This was supported in another study that examined multiple forms of HRV data collection and in non-stationary conditions wrist derived PPG misses large sections of data [18]. This points to the need for wrist-worn solutions to include an accelerometer to provide a measure of motion, which can be used as a factor to determine if a detected beat should be considered valid and included in the data.
Long-term monitoring of HRV and wearable technology have the potential to give medical providers new insights into mental health that has not yet seen the rise in technology for diagnosis and treatment as other medical conditions have. Studies have shown that HRV is significantly altered in patients with a history of depression [1]. The overall mechanism for this is the understanding of the autonomic nervous system, in particular the dysregulation of the parasympathetic and sympathetic nervous system. This has also shown promise in empowering patients through biofeedback to guide therapies for anxiety and stress [19]. This is further supported by off-the-shelf, wearable wrist PPG showing good accuracy with HRV metrics and the ability to detect changes when subjects were put through stressful exercises compared to non-stress activities [20]. Further research will need to focus on various time durations of monitoring as this can be significantly impacted by motion artifact with wrist movement.
Limitations
There are a number of limitations in this study. This was a small cohort of patient tracings. We selected a small cohort because we wanted gold-standard PPG tracings. However, larger studies may be warranted to confirm these findings. In addition we used a cutoff of a mean absolute percent difference as our outcome. This was a somewhat arbitrary cutoff, but within the 5% error that is generally considered clinically meaningful. A final limitation of this study was the short time frame of data acquisition. The one-minute data segments impact the ability of low-frequency HRV analysis as this generally requires longer tracings. However, the one-minute data segments allow for better chances of clean data segments during non-stationary conditions as it is much more likely to capture one minute of clean, artifact-free data, rather than five minutes or greater.
Conclusions
Therefore research aimed to identify the optimal inflection point above which removing a percentage of beat to beat intervals or slightly selecting the peak at the wrong sample can guide HRV analysis in non-stationary conditions. This study shows that time domain metrics including RMSSD and SDNN are the most robust measures that can tolerate missing or shifting data, while pNN50 and frequency-domain indices appear to be most sensitive to these changes.
Acknowledgements
None.
Notes
The authors have no potential conflicts of interest to disclose.
Author Contributions
Conceptualization: David C. Sheridan, Ryan Dehart, Steven D. Baker. Data Curation: David C. Sheridan, Michael Sabbaj, Ryan Dehart, Steven D. Baker. Formal analysis; Amber Lin. Writing—original draft: David C. Sheridan. Writing—review and editing: all authors.