Prevalence of Addictive Behaviors in Medical Students and Their Association With Stress
Article information
Abstract
Objective
This study aims to investigate the prevalence of the addictive use of the internet, smartphone, and alcohol in medical students, the association of this addictive use with stress, and the mediating roles of resilience and self-esteem in this association.
Methods
A total of 866 medical students completed measures of three addictive uses as well as psychological scales for stress, resilience, and self-esteem. Correlation analyses and parallel mediation analysis were carried out.
Results
The prevalence of potential-risk and high-risk users was 5.8% and 1.7% for internet use, 5.4% and 2.2% for smartphone use, 22.6% and 5.3% for alcohol use, respectively. All three addictive behaviors tended to increase in terms of prevalence or mean score according to an increase in a students’ grade. Stress was positively correlated with internet use (r=0.324, p<0.001) and smartphone use (r=0.347, p<0.001). Resilience and self-esteem were found to be mediators in the association between stress and internet use or smartphone use.
Conclusion
These findings suggest that addictions in medical students are as prevalent as in a general population and that internet use and smartphone use may be better explained by a stress-addiction model with resilience and self-esteem as mediators than alcohol use.
INTRODUCTION
Since university students are important resources who will take charge of future leading roles and positions in society, their mental health has been regarded as an important aspect in the development of individuals and society [1]. Due to the current trend in which university courses tend to be considered as stepping stones to success rather than be chosen based on student’s abilities and aptitudes, university students are under a lot of stress due to excessive competition and preparation for study and employment [2,3]. Medical students tend to have less stress about employment or anxiety about the future than their non-medical academic peers, but their academic stress is severe due to excessive learning and relative sense of failure among their peers. They also have high level of anxiety due to repetitive tests, extreme competition, and flunk system. Therefore, mental problems may occur more frequently in medical students than those in the general population [4]. In particular, medical students have experienced addiction as well as depression, anxiety, and suicidal ideation [5-8]. Since the mental health of medical students is associated with the quality of health care in the future [9], it is essential to propose an intervention strategy for the prevention and treatment of these conditions.
Previous studies have investigated the prevalence of high-risk and potential-risk users of each addictive behavior in medical students. For risky users, the prevalence ranged from 5.6%–52.4% for internet use, 4.4%–60.3% for smartphone use, and 15.4%–45.5% for alcohol use [5,10-14]. Some prevalence values seem high relative to the prevalence of the general adult population [15,16]. However, these studies most reported only one or two types of addiction and did not show overall pattern of addiction across several types of addictive behavior. Moreover, only a few studies have compared the levels of addiction among medical students by grade, mostly due to small study sample sizes.
Stress is a known risk factor for the development of addiction [17]. From a biological perspective, stress increases an individual’s vulnerability to addiction through mechanisms, such as chronic stress-mediated changes in the dopaminergic system [17]. Also, stress affects the catecholaminergic modulation of prefrontal circuits, which in turn impairs executive functions such as inhibitory processes, which are associated with self-control and impulse control.18 From a psychological perspective, impaired coping skills and an increased sensitivity to negative emotions also increase an individual’s vulnerability to addiction [17,18].
As such, many studies have compared the association between addictive behavior and stress, but only a few studies have been conducted on medical students. Among them, studies on internet use and smartphone use have shown a positive correlation between stress and addictive behaviors, but studies on alcohol use are not clear, as they show either negative correlation or no correlation [5-8,19,20]. Although more studies are needed, these mixed findings may indicate that many internal and external factors are involved in the relationship between stress and addiction. The current study focused on the protective psychological factors, specifically psychological resilience and self-esteem that can reduce addictive behavior.
Psychological resilience refers to effective coping and adaptation to adverse situations in a positive manner [21,22]. Resilient individuals use positive emotions to alleviate the effects of stress and show physiological differences in their capability to adapt to stress [21]. It was also found that the higher the level of a participant’s resilience, the lower the level of internet addiction [22]. On the other hand, self-esteem is defined as a global barometer of self-evaluation that involves a cognitive appraisal about general self-worth and affective experiences of the self [23]. A high level of self-esteem equips individuals with the abilities to handle unpleasant situations, cope effectively with challenges, and positively mediate the expression of dysfunctional schema when negative life events are experienced. Previous studies found a significant correlation between self-esteem and internet addiction in university students as well as a mediating effect of self-esteem between stress and substance abuse among adolescents [24,25]. To the best of our knowledge, despite the obvious relationships between addictive behaviors, stress, and resilience or self-esteem, no studies have been conducted on the mediating roles of resilience and self-esteem in the relationship between stress and various addictive behaviors among medical students.
Therefore, the aim of this study is to simultaneously investigate the levels of internet addiction, smartphone addiction, and alcohol addiction in a relatively large number of medical students and to determine differences according to grades. In addition, this study aims to investigate the relationship between stress and addictive behaviors, as well as the roles of resilience and self-esteem as mediating factors.
METHODS
Research design and sample
The Kyungpook National University School of Medicine conducted mental health assessments on students annually from 2009, and the data used in this study were drawn from three grades (premedical first grade, medical first grade, medical third grade) from 2017 to 2020. The students responded to self-report questionnaires through a computer-based test. In the case of the results of the subjects who performed the tests more than once as follow-up observations, only the first test results were analyzed.
In proportion to the sex distribution of all medical students, the data of 866 students, consisting of 613 men (70.8%) and 253 women (29.2%), were included in the final analysis. According to the distribution by grade, 356 (41.1%) were first-year premedical students, 297 (34.3%) were first-year medical students, and 213 (24.6%) were third-year medical students.
This study was approved by the Institutional Review Board of Kyungpook National University School of Medicine (IRB No. 2021-0086). All of the subjects were fully informed about the aims and methods of the study, participated voluntarily, and gave written informed consent prior to their participation in this study.
Measurement of addiction
Internet addiction
The short form internet addiction proneness scale for adult: self-report, developed by the National Information Society Agency, was used to measure internet addiction (Cronbach’s alpha [α]=0.87) [26]. It consists of a total of 15 questions, and each item is evaluated with a score from 1 (not at all) to 4 (extremely). The question is composed of four sub-factors: daily life impairment, virtual world orientation, withdrawal, and tolerance. In accordance with grading standards, the sample was classified into three groups according to the level of internet addiction measured: high-risk user groups, potential-risk user groups, and general user groups [26]. A high-risk user group is one that has a total score of 42 or higher or that meets all 14 or more points of daily living disability, 12 or more points of withdrawal, and 13 or more points of tolerance. A potential-risk user group has a total score of 39 to 41 points or 14 or more points of daily living disability. A general user group is a group that does not belong to either of the other two groups.
Smartphone addiction
To measure smartphone addiction, we used the Korean smartphone addiction proneness scale for adults, which was developed by the National Information Society Agency (Cronbach’s α=0.81) [27]. Each of the sub-factors, including daily life impairment, virtual world orientation, withdrawal, and tolerance, consists of a total 15 questions and is rated from 1 (not at all) to 4 (extremely) points. The sample was classified into three groups according to the scoring criteria of the National Information Society Agency. A high-risk user group is one that has a total score of 44 or higher or that meets all 15 or more points of daily living disability, 13 or more points of withdrawal, and 13 or more points of tolerance. A potential-risk user group has a total score of 40 to 43 points or 14 or more points of daily living disability. In addition, a general user group is a group that does not belong to either of the other two groups.
Alcohol addiction
The Alcohol Use Disorders Identification Test (AUDIT), which was developed by the World Health Organization (WHO) to screen dangerous drinkers, was used to measure the levels of alcohol addiction among the students. The scale consists of 10 questions regarding drinking behaviors (3 questions), dependence symptoms (3 questions), and alcohol-related problems (4 questions) and is evaluated on a scale of 0 to 4 points. The Korean version of AUDIT is based on the amount of Korean liquor and has been validated on the Korean population (Cronbach’s α=0.92) [28]. According to the Korean version standard [29], men who scored more than 20 points as well as women who scored more than 10 points were classified into the high-risk drinking group (the supposed alcohol use disorder group), and men who scored between 10 and 19 points as well as women who scored between 6 and 9 points were classified into the at-risk drinking group. In addition, a general user group is a group that does not belong to either of the other two groups.
Measurement of stress, resilience, and self-esteem
The Perceived Stress Scale (PSS) is an instrument used to measure the level of stress experienced over the past month. In this study, we used the Korean version of the PSS, which has a Cronbach’s α value of 0.82 [30]. A total of 10 questions are evaluated along a scale of 0 (never) to 4 (very often) points. Questions 4, 5, 7, and 8 are negative questions and are back-scored. The total range is 0 to 40 points. The higher the total score, the greater the perceived level of stress.
The Connor-Davidson Resilience Scale (CD-RISC) is used to evaluate resilience related to coping skills in stressful events. The Korean version of the CD-RISC features excellent internal consistency and was developed in 2010 (Cronbach’s α>0.9) [31]. It consists of a total of 25 questions, and each item is evaluated with a score of 0 (not at all) to 4 (extremely) points. The scores of all items are summed, with a higher score indicating greater psychological resilience.
The Rosenberg Self-Esteem Scale is a measurement that evaluates an individual’s self-esteem, which is the level to which one respects oneself and the level to which one considers oneself worthwhile. A total of 10 questions are evaluated with a score of 0 (not at all) to 3 (extremely) points, and Questions 3, 5, 8, 9, and 10 are scored inversely. Higher scores indicate higher self-esteem. In this study, the scale tool which was developed by Rosenberg and cited by Yoo et al. [32] was used (Cronbach’s α=0.86).
Statistical analysis
For each addictive behavior (internet, smartphone, and alcohol use), we used Pearson’s chi-square test to compare the sex and grade-level differences in the prevalence of high-risk and potential-risk groups. A one-way analysis of variance was used to compare the differences in the levels of the addictive behaviors and psychological variables (stress, resilience, and self-esteem) among the three groups (premedical first grade, medical first grade, medical third grade). The same differences were also tested between male and female students by means of a t-test.
To analyze the relationship between addictive behaviors and psychological variables, zero-order and partial correlation analyses with sex and grade corrections were performed. For the purpose of correcting for multiple testing, the Bonferroni correction was applied to statistical significance calculations.
Since stress, resilience, and self-esteem are overlapping and correlated concepts, various mediation models need to be considered. However, this study focused on the roles of resilience and self-esteem as mediators in the relationship between stress and addictive behaviors, based on a previously confirmed mediation model [25]. The effects of self-esteem and resilience as mediators of the association between stress and addictive behaviors were analyzed via a parallel mediation model after controlling for sex and grade. This analysis was completed with the Statistical Package for Social Sciences (SPSS) PROCESS macro, version 3.5 (model 4). The bootstrapping method was used in this analysis. The calculated confidence intervals (CIs) were used to determine whether each effect (i.e., direct, indirect) was statistically significant. For each effect, we examined the 95% CI and if the value of 0 did not fall within the range of the CI for that effect, then the effect was deemed to be statistically significant.
We performed all statistical analyses using SPSS, version 20.0 (IBM Corp., Armonk, NY, USA). For these analyses, a two-sided test at the 0.05 level was used to evaluate statistical significance.
RESULTS
Prevalence of internet, smartphone, and alcohol addiction
Table 1 represents the prevalence of addiction in internet, smartphone, and alcohol use. Among the entire sample of students, the prevalence of potential-risk and high-risk users was 5.8%, and 1.7% for internet use, and 5.4% and 2.2% for smartphone use, respectively. Moreover, for alcohol use, the prevalence was higher than that of the other addictive behaviors, with the prevalence of at-risk drinking group being 22.6% and the prevalence of the high risk-drinking group being 5.3%.

Prevalence of risky internet, smartphone, and alcohol users by grade and sex among medical students (N=866)
In terms of grade, the risky internet users, who met the high-risk or potential-risk categories, increased from 4.8% to 8.4% to 10.8% as they were going up in grade (χ2=7.5, p=0.023). The risky alcohol users also tended to increase from 23.9% to 28.3% to 34.3% (χ2=7.18, p=0.028). In contrast, the number of risky smartphone users showed no statistical difference according to grade. Additionally, there was no sex difference in the prevalence of risky users of the internet and smartphone, while the prevalence of risky alcohol users was higher in females than in males (χ2=13.79, p<0.001).
Differences between quantitative scores of addictive behaviors and psychological variables
Table 2 shows the quantitative differences by grade and sex for each addiction behavior. For grade-level differences, the first-year premedical students showed significantly lower scores on internet and smartphone use than both the first and third-year medical students did. However, there was no grade-level difference in alcohol use. Regarding the difference between the sexes, males reported higher scores than females in the internet and alcohol use but not in the smartphone use.
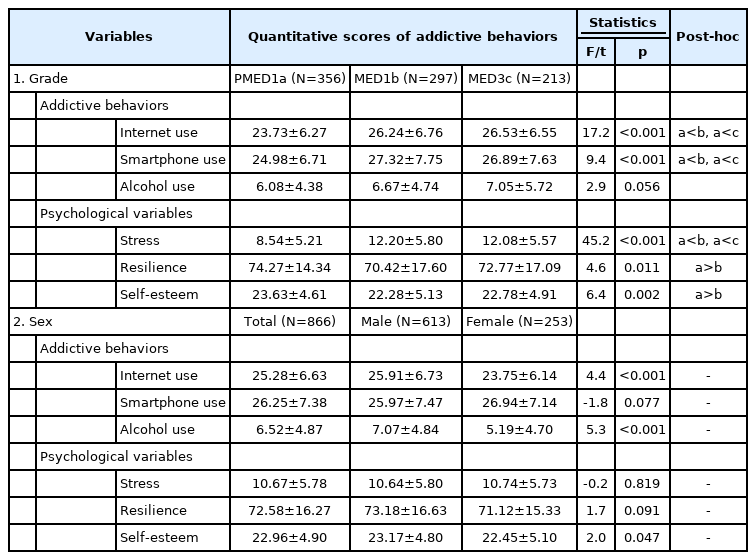
Differences in quantitative scores of addictive behaviors and psychological variables by grade and sex among medical students (N=866)
Among psychological variables, perceived stress in the first-year premedical students was significantly lower than that in observed the other two grades, whereas resilience and self-esteem in the first-year premedical students were higher than those observed in the first-year medical students. Meanwhile, self-esteem was the only variable that showed a significant sex difference, with scores being higher in males than in females.
Correlations of stress, resilience, and self-esteem with addictive behaviors
Table 3 shows the correlations between the psychological variables and the addictive behaviors. Stress was positively correlated with internet use and smartphone use (all Ps<0.001). Resilience was negatively correlated with internet use and smartphone use (all Ps<0.001). Self-esteem was also negatively correlated with internet use and smartphone use (all Ps<0.001). These relationships remained significant after controlling for sex and grade. However, the level of alcohol use was not correlated with stress, resilience, or self-esteem, regardless of whether sex and grade were controlled.
The mediating effect of resilience and self-esteem on the association between stress and addictive behaviors
The regression model for each addictive behavior was found to be statistically significant (all Ps<0.001). In addition, for the analysis of statistical power, the R2 values associated with the F-tests of the addictive behavior models were: in the internet use model, 0.27 (self-esteem as the outcome), 0.16 (resilience as the outcome), and 0.25 (internet use as the outcome); in the smartphone use model, 0.27 (self-esteem as the outcome), 0.16 (resilience as the outcome) and 0.24 (smartphone use as the outcome); and in the alcohol use model, 0.27 (self-esteem as the outcome), 0.16 (resilience as the outcome), and 0.05 (alcohol use as the outcome). According to the standard for R2 values proposed by Cohen [33], the statistical power of our study is above the medium level (R2=0.13), with the exception of the alcohol use model.
The mediating effects of resilience and self-esteem on stress and each addiction behavior were different (Table 4). For internet use, resilience and self-esteem mediate the relationship between stress and internet use. In addition, the sum of the indirect effects was 0.20, which was statistically significant, as the 95% CI (0.15–0.24) did not include 0. For smartphone use, a statistically significant indirect effect of stress on smartphone use was revealed through resilience but not through self-esteem. Moreover, the sum of the indirect effects was 0.18, which was statistically significant, as the 95% CI (0.13–0.24) did not include 0. On the other hand, for alcohol use, the 95% CIs for the indirect effect contained 0, suggesting that neither resilience nor self-esteem was a significant mediator on the effect of stress on alcohol use. Figure 1 depicts the summary of the relationship between stress, resilience, self-esteem, and each addiction behavior.
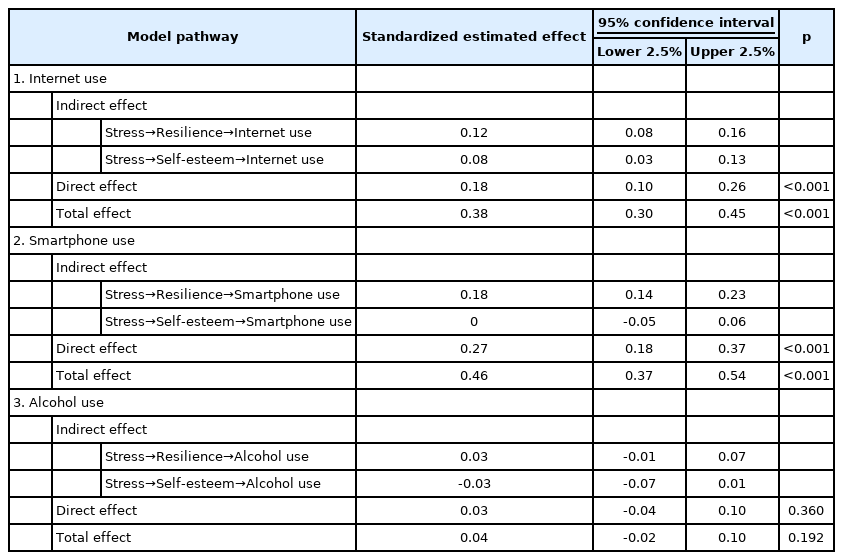
Mediating effect of resilience and self-esteem on the association between stress and addictive behaviors
DISCUSSION
We investigated the prevalence of three addictive behaviors and their associations with stress in medical students. The prevalence of potential-risk and high-risk users was, respectively, 5.8% and 1.7% for internet use, 5.4% and 2.2% for smartphone use, and 22.6% and 5.3% for alcohol use. All three addictive behaviors tended to increase in terms of prevalence or mean score with increasing grade levels. In addition, there were associations between internet and smartphone use and stress, in which resilience and self-esteem acted as mediators. However, the association between stress and alcohol use, and the mediating roles of them on this association were not found in alcohol use.
Prevalence of risky internet, smartphone, and alcohol users
In the literature, the prevalence of addictive behaviors of medical students varies greatly from study to study [5,10-14]. For risky users, the prevalence was 5.6%–52.4% for internet, 4.4%–60.3% for smartphones, and 15.4%–45.5% for alcohol [5,10-14]. These discrepancies between studies may be due to differences in the measurement scale (including cut-off values), the educational environments, the sample size and distribution, and the socio-cultural backgrounds. However, the prevalence of risky internet use and alcohol use in our study seems to be similar to that of the Korean general adult population in other surveys using the same measurement scale (internet use: 5.8%, and alcohol use: 33.2%) [15,16]. Thus, the prevalence in this study is indicative of potential public health concerns regarding addictive behaviors among medical students.
Grade-level differences of addictive behaviors and psychological variables
In this study, qualitative and quantitative analyses demonstrated characteristic changes of use pattern among three grades. The mean scores of internet and smartphone use were higher in the first and third graders of the medical school than in the first graders of the premedical school. These findings indicated that the general use of the internet and smartphone was increased during this period. In contrast, an increase in the proportion of risky users was not observed in smartphone use, though it was in internet use, as grade increased.
The first graders of the premedical school who took the painful entrance exam enjoyed the joy of experienced entering medical school, so their level of stress were low, and their internet and smartphone use was believed to be less than that of other grades [20]. In fact, consistent with previous study [34], perceived stress was found to be higher in the first and third graders of the medical school than in the first graders of the premedical school in this study. These observations support the notion that the internet and smartphone are used as means by which to reduce stress from negative emotions [35]. Another explanation of the general increase of internet and smartphone use may be due to heightened accessibility, as university students can access the internet and smartphone more freely than high school students can. Third, social influences should also be considered. Internet use and smartphone use (being connected online) have become social norms, and there are expectations of constant reachability [36]. According to Erikson’s developmental stages, the developmental task of early adulthood is to expand the sense of intimacy by forming meaningful interpersonal associations with others while increasing interest in others [37]. In this process of psychosocial development, it is a process in which the use of the internet or smartphone itself reacts to social conformity as a result of peer pressure [38]. In addition, based on the social learning theory, the use of the internet and smartphone can also be viewed as a process of adapting to social life by modeling social surroundings [39]. Therefore, in the process of adapting to university life, it can be considered that the use of the internet and smartphone would gradually increase. Although both internet and smartphone use increased, the prevalence of risky users only increased with respect to internet use. We assume that risky internet users may include internet users for entertainment purpose, such as multi-user online games or gambling games, which may be more addictive than smartphone [40]. Future studies are needed to see what kinds of contents lead risky internet users.
On the other hand, although no significant differences in the mean scores of alcohol use were found, risky alcohol users increased from 23.9% to 28.3% to 34.3% as grade level increased, and this prevalence was higher than that of other addictive behaviors in this study. Unlike internet and smartphone use, alcohol did not show generally increased use according to grade partially because exposure to alcohol is less frequent than internet and smartphone use. Nevertheless, the reason why the proportion of risky alcohol users increases is that alcohol addiction may be more biologically susceptible than the other addiction behaviors. Last but not least, it should be noted that there were no statistical differences between the first and third medical students in the mean scores of all addictive uses and psychological variables in this study.
Sex differences of addictive behaviors
Consistent with previous studies [40], males generally reported to use the internet more than females in this study. However, there was no difference in the prevalence of risky users. These results could be explained by differences in the internet usage preferences of males and females. Whereas females prefer social network services, males prefer to use the internet for entertainment purposes, such as multi-user online games, which may be more addictive [40]. For smartphone use, there were no sex differences in general use or in the prevalence of risky users. Regarding alcohol use, the mean score of females was lower while the prevalence in the risk drinking group is higher in females than in males. Interpretation of these results should be cautious because the cutoff value of risky drinking is lower in for females than for males. However, this difference may not come solely from different cutoff values. In fact, the sex gap in the prevalence of alcohol use disorder has been rapidly decreasing over time in South Korea. In particular, the prevalence of alcohol use disorder among young women is increasing [41], which may be indicative of a phenomenon caused by an increase in women’s social advancement and socio-cultural changes, such as the shift to a more permissive drinking culture.
Associations between stress and internet, smartphone, and alcohol use and the mediating effects of resilience and self-esteem
There were significant correlations between stress and internet use and smartphone use, and these associations were well shown in regression analysis. Stress is thought to play a role in the development of addiction by increasing craving and motivation toward the addictive behavior [42]. The association between stress and addictive behaviors may be bidirectional; such addictive behaviors may be used as a means of reducing stress, and blunted stress responses may confer an increased risk of engaging in addictive behaviors [42].
On the other hand, in the regression analysis, there were no association was observed between alcohol and stress as in the previous study [7], and this was the same in correlation analysis. Based on studies showing that alcohol is used as a kind of countermeasure to unpleasant things or situations and that alcohol has the effect of self-treatment in buffering stress in life [43], it was thought that stress affected alcohol use. However, from a neurobiological point of view, alcohol (substance addiction) directly affects the dopamine system of the brain, whereas behavioral addictions only indirectly affect these systems [44]. Also, alcohol, unlike behavioral addiction, is accompanied by a physiologically distinct and medically serious withdrawal condition [44]. In these respects, alcohol could be considered to have a greater effect on addiction. Moreover, alcohol use seems to be more genetically innate than behavioral addictions (e.g., internet use) [45]. Therefore, it could be considered that alcohol causes addiction through a much more complex and diverse pathway that stress alone cannot explain.
Aside from directly affecting addictive behaviors, stress also had indirect effects through resilience and self-esteem in this study. That is, resilience acted as mediating role with respect to the effect of stress on internet use and smartphone use, and self-esteem acted as mediating role with respect to the effect of stress on internet use. The medical students who perceived high stress had lower resilience or self-esteem, resulting in higher levels of addictive behaviors, while those who perceived low stress had higher resilience or self-esteem, contributing to lower levels of addictive behaviors. Resilience and self-esteem were associated with positive emotions, which protect mental health by controlling the negative effects of stress [46,47]. Moreover, it was found that people with high resilience and people with high self-esteem possess many psychological resources, including tranquility, optimism, and openness [46,48], and are more likely to find positive meaning in problems that they have to face [46,48]. Based on the protective factor model [49], these positive personal attributes reduce the negative influence of stress on adaptive behaviors. Thus, people with high resilience and people with high self-esteem are less likely to engage in addictive behaviors.
Implication of mental health for medical students
According to the results of this study, it would be helpful to provide premedical students with education focused on the primary prevention of addiction. For junior medical students who go through drastic changes in their learning systems, the management of their academic performance and its associated stress is critical. For senior medical students, school authorities need to focus more on the detection and treatment of students at risk because we observed that there were no general increases in addictive behaviors between first and third grade medical students. Moreover, intervention strategies to enhance resilience and self-esteem should be considered, especially with respect to internet and smartphone use, as the improvement of resilience and self-esteem is crucial in alleviating maladaptive coping and in further promoting coping mechanisms [48], resulting in lower levels of addictive behaviors.
This study has several limitations. First, since our study was a cross-sectional study, we were unable to determine definite causal relations among stress, resilience or self-esteem, and addictive behaviors. Second, the data were obtained through self-reported questionnaires, which could lead to response bias. Third, the ratio of male and female students in this study was approximately 7:3. Although it was proportional to the proportion of the total student distribution, there were a possibility of selection bias. Thus, we have tried to control sex as a covariate in the correlation analysis and mediation analysis. Fourth, due to a lack of data on other variables, including living status, academic performance, and psychological symptoms, the relationships between these factors and addictive behaviors were not considered. Fifth, since this study focused on students from a single medical school, we should be careful in generalizing our results. Further prospective studies of medical students of all grades, including those attending other universities, are needed to compensate for our limitations. Nevertheless, our study has the following strengths. For the first time, we investigated the prevalence of various addictive behaviors by grade and their associations with stress through resilience and self-esteem on various addictive behaviors in Korean medical students.
In conclusion, our study suggests that addictions in medical students are as prevalent as among the general population and that the prevalence may increase with increasing grade in medical school. We also suggest that internet use and smartphone use may be better explained by stress than alcohol use, with resilience and self-esteem acting as mediators in their relationships. This study may provide valuable information for the relevant university and medical school authorities responsible for managing addictive behaviors in medical students.
Notes
Availability of Data and Material
The datasets generated or analyzed during the study are available from the corresponding author on reasonable request.
Conflicts of Interest
Seung Jae Lee, a contributing editor of the Psychiatry Investigation, was not involved in the editorial evaluation or decision to publish this article. All remaining authors have declared no conflicts of interest.
Author Contributions
Conceptualization: Jimin Lee, Seunghee Won, Seung Jae Lee. Data curation: Jimin Lee, Byung-Soo Kim, Sung Man Chang. Formal analysis: Jimin Lee, Seung Jae Lee. Investigation: Byung-Soo Kim, Sung Man Chang, Seunghee Won. Methodology: Jimin Lee, Seung Jae Lee. Writing—original draft: Jimin Lee, Seung Jae Lee. Writing—review and editing: Seung Jae Lee, Sung Man Chang. Approval of final manuscript: all authors.
Funding Statement
None